Announcing Strathweb Phi Engine - a cross-platform library for running Phi-3 anywhere
I recently wrote a blog post about using Rust to run Phi-3 model on iOS. The post received an overwhelmingly positive response, and I got a lot of questions about running Phi-3 using similar approach on other platforms, such as Android, Windows, macOS or Linux. Today, I’m excited to announce the project I have been working on recently - Strathweb Phi Engine, a cross-platform library for running Phi-3 (almost) anywhere.
Built-in support for Server Sent Events in .NET 9
Many years ago I wrote a book about ASP.NET Web API. One of the chapters in that book was dedicated to supporting push communication between the server and the client, and one of the covered techniques was the niche technology called Server-Sent Events (SSE). At the time, SSE was not widely supported by browsers, however, it was super simple and effective way to push data from the server to the client, and it was a great fit for scenarios where you needed to push data from the server to the client in a one-way fashion without much ceremony.
Over the years, SSE has not really gained much traction, and WebSockets have become the de-facto standard for push communication. However, in recent years a certain OpenAI came out with their API that uses SSE for streaming responses from their Large Language Model, and, pretty much overnight, SSE became cool again.
In .NET 9, SSE finally is getting first-class client-side support and the first preview was released this week with .NET Preview 9.
Announcing Q# Bridge - a library bringing the Q# simulator and tools to C#, Swfit and Kotlin
Over the past year, Q# and the QDK, have undergone a massive transformation, with the entire toolchain moving to Rust - which resulted in a significant performance improvement, better portability of the toolchain and the ability to run Q# on a wide range of platforms. This was especially striking compared to the 0.x versions of the QDK, which was coupled to the .NET SDK.
Today I would like to announce a new Q# ecosystem project called Q# Bridge, built under the Q# Community organization, which acts as a wrapper around the Q# simulator and tools, allowing you to easily use them from C#, Swift and Kotlin. The library is designed to be a lightweight wrapper around the Q# tooling, providing a simple API to interact with the Q# simulator and tools (such as resource estimation, QIR generation or circuit descriptions), without the need to write any Rust code or deal with marshaling or FFI directly.
Running Microsoft's Phi-3 Model in an iOS app with Rust
Last month, Microsoft released the exciting new minimal AI model, Phi-3 mini. It’s a 3.8B model that can outperform many other larger models, while still being small enough to run on a phone. In this post, we’ll explore how to run the Phi-3 model inside a SwiftUI iOS application using the minimalist ML framework for Rust, called candle, and built by the nice folks at HuggingFace.
Tool Calling with Azure OpenAI - Part 2: Using the tools directly via the SDK
Last time around, we discussed how Large Language Models can select the appropriate tool and its required parameters out of freely flowing conversation text. We also introduced the formal concept of those tools, which are structurally described using an OpenAPI schema.
In this part 2 of the series, we are going to build two different .NET command line assistant applications, both taking advantage of the tool calling integration. We will orchestrate everything by hand - that is, we will only use the Azure OpenAI Service API directly (or rather using the .NET SDK for Azure OpenAI) - without any additional AI frameworks.
Tool Calling with Azure OpenAI - Part 1: The Basics
One of the fantastic capabilities of the Large Language Models is their ability to choose (based on a predefined set of tool definitions) the appropriate tool and its required parameters out of freely flowing conversation text. With that, they can act as facilitators of workflow orchestration, where they would instruct applications to invoke specific tools, with specific set of arguments.
OpenAI announced the built-in capability called function calling in the summer of last year, and by now it is an integral part of working with and building applications on top of the GPT models. The functionality was later renamed in the API to “tools”, to better express their broad scope and nature.
Today I am starting a new multi-post Azure OpenAI blog series focusing specifically on the tool capabilities. We will build a client application with .NET, and explore tool integration from different angles - using the Azure OpenAI .NET SDK directly, using the Assistants SDK and finally leveraging various orchestration frameworks such as Semantic Kernel and AutoGen. In today’s part one, we are going to introduce the basic concepts behind tool calling.
Combining Azure OpenAI with Azure AI Speech
In my recent posts, I’ve been exploring various facets of the Azure OpenAI Service, discussing how it can power up our applications with AI. Today, I’m taking a slightly different angle - I want to dive into how we can enhance our projects further by integrating Azure OpenAI Service with Azure AI Speech. Let’s explore what this integration means and how it could lead to exciting, AI-powered applications.
Using your own data with GPT models in Azure OpenAI - Part 4: Adding vector search
For our Retrieval-Augmented-Generation (RAG) application, we setup AI Search in part 1, however so far we only used it using the basic keyword search.
In this part 4 of the series about bringing your own data to Azure OpenAI Service, we will go ahead and integrate vector search, as a more sophisticated way of performing the search across the Azure AI Search index within our RAG-pattern system.
I already covered vectorization and embeddings using the OpenAI embedding model on this blog, and we will be relying on the same principles here. I recommend reading through that article before continuing if you are not yet familiar with the concept of embeddings.
Running Swift Package tests in Azure DevOps
It is a common scenario to use Azure DevOps to build, sign and release iOS applications. Most of the tasks related to that can be handled by the Xcode@5 task, which provides support for all kinds of build activities around Xcode workspaces, and which is a de-facto shortcut for invoking xcodebuild command line tool.
The task is quite well documented, but it is not entirely obvious how to use it for building Swift Packages, as those come without Xcode workspaces. In this post we will quickly explore how to achieve that.
Upgrading Q# book samples to QDK 1.0
As you may know from the announcement on this blog (or from the image in the sidebar…), I wrote a quantum computing book titled “Introduction to Quantum Computing with Q# and QDK” which was published by Springer in May 2022. The source code samples used in the book were written against version 0.21.2112180703 of the QDK and the Q# language, which had been released on 14th December 2021. The code also works fine with all the newer versions of QDK lower than 1.0 - the last pre-1.0 release being 0.28.302812, from 15 September 2023.
Earlier this month, Microsoft announced the release of QDK 1.0, which brings in a big overhaul of the entire QDK ecosystem, as well as the Q# language itself. As a consequence, it also contains numerous breaking changes and feature gaps in the libraries and in the language itself.
As part of my commitment to keeping the samples used in the book up-to-date, I would like to share the updated samples.
About
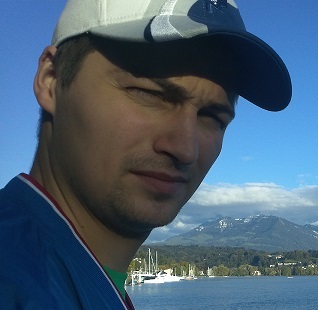
Hi! I'm Filip W., a cloud architect from Zürich 🇨🇭. I like Toronto Maple Leafs 🇨🇦, Rancid and quantum computing. Oh, and I love the Lowlands 🏴.
You can find me on Github and on Mastodon.
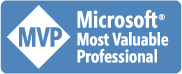
Recent Posts
- 2024/07/25, Announcing Strathweb Phi Engine - a cross-platform library for running Phi-3 anywhere
- 2024/07/12, Built-in support for Server Sent Events in .NET 9
- 2024/06/25, Announcing Q# Bridge - a library bringing the Q# simulator and tools to C#, Swfit and Kotlin
- 2024/05/09, Running Microsoft's Phi-3 Model in an iOS app with Rust
- 2024/04/19, Tool Calling with Azure OpenAI - Part 2: Using the tools directly via the SDK
Categories
- ai (11)
- ai search (4)
- apache cordova (1)
- asp.net 5 (17)
- asp.net core (45)
- asp.net mvc (35)
- asp.net mvc 6 (7)
- asp.net vnext (6)
- asp.net web api (96)
- astronomy (1)
- azure (19)
- azure service bus (1)
- azure-devops (1)
- benchmark dotnet (1)
- bing maps (1)
- blazor (1)
- c plus (2)
- c-sharp (154)
- cryptography (3)
- csharp (4)
- csharp 10 (2)
- dnx (3)
- dotnet-cli (2)
- dotnet-script (11)
- duende (2)
- editorconfig (1)
- entity framework (2)
- espn api (2)
- events (1)
- ffi (4)
- fsharp (1)
- git (1)
- glimpse (1)
- html5 (4)
- identity server (2)
- iis (2)
- il (1)
- intro to qc (19)
- ios (4)
- javascript (9)
- jquery (4)
- jquery mobile metro (1)
- katana (2)
- kindle (1)
- knockout.js (8)
- kotlin (2)
- last.fm api (2)
- linq (1)
- mac (2)
- macos (1)
- mathematica (1)
- msbuild (3)
- mvc core (3)
- nancy (2)
- native (1)
- net (141)
- net 5 (3)
- net 6 (5)
- net 7 (7)
- net 8 (3)
- net 9 (1)
- net core (49)
- net sdk (2)
- ninject (2)
- odata (4)
- oidc (2)
- omnisharp (13)
- openai (9)
- osx (2)
- owin (5)
- php (1)
- q-sharp (34)
- qir (3)
- quantum computing (37)
- roslyn (30)
- rust (4)
- scriptcs (11)
- scripting (9)
- security (6)
- servicestack (2)
- signalr (7)
- swift (7)
- testing (5)
- twitter boostrap (1)
- typescript (1)
- visual studio (4)
- visual studio code (11)
- wasi (3)
- wasm (3)
- windows phone 7 (1)
- wordpress (1)
- wpf (2)